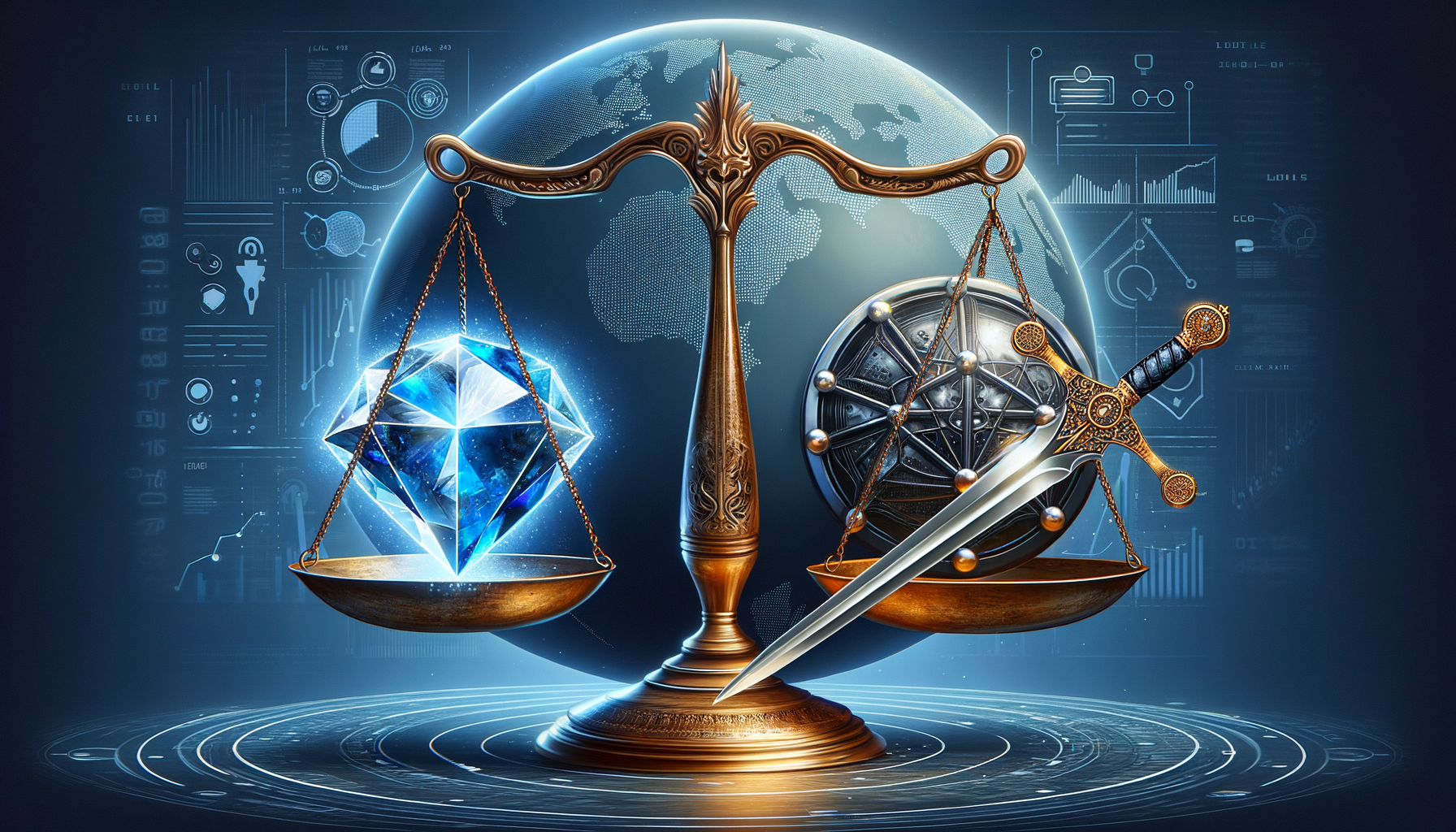
Artificial intelligence is ushering in the next renaissance of human innovation and progress. The incredible potential AI holds to revolutionize fields like healthcare, education, scientific research, renewable energy, and more is staggering. However, like many transformative technologies before it, AI also carries grave risks if weaponized and directed towards nefarious aims.
As AI systems become more advanced and powerful, keeping this world-altering technology out of the wrong hands represents one of the preeminent security challenges of our time. Allowing terrorist organizations or rogue states to leverage AI could enable a nightmarish array of potential attacks and disruptions impacting entire societies.
This report will explore the major areas of concern around AI terrorism, analyze specific risks and scenarios, and propose multi-layered solutions to safeguard AI as a force for good rather than destructive ends. Policymakers, AI developers, security professionals, and engaged citizens all have critical roles to play.
The AI Terrorism Threat Matrix
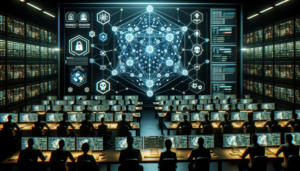
The dangers of AI terrorism span a wide gamut of potential attacks and disruptions across both physical and digital battlefields. Here are some of the highest-risk areas of concern:
AI-Enabled Cyber Attacks & Security Breaches
Malicious cyber actors have already caused billions in damages by hijacking corporate networks, stealing sensitive data, and holding systems for ransom. AI vastly amplifies their capabilities for penetrating network defenses and evading detection.
AI systems can be used to automatically scan for software vulnerabilities at scales impossible for human hackers. Their superior pattern recognition allows AI to pinpoint subtle flaws in code that people would easily miss. AIs can also dynamically morph their attacks and shift strategies in real-time based on a target’s responses.
Once inside a network, AI malware could rapidly pivot and escalate attacks across the whole system before defenders can react. AI-driven password guessing and authentication cracking would also become trivial exercises. Nasty strains of AI malware may become self-replicating and self-modifying to continually evolve and survive remediation attempts.
Beyond generic cyber mayhem, advanced AI agents could be directed onto specific high-value targets like power grids, air traffic control systems, banking networks, and other civilian critical infrastructure to trigger devastating disruptions or hijack control. The nightmare scenario of AI software burrowing into our infrastructure and lying dormant for years before initiating an attack on command is a clear national security threat.
Autonomous Weapons & Drones
AI technologies are already playing important roles in drone targeting and munitions guidance for modern militaries. Of grave concern is the potential development and deployment of autonomous weapons systems that can identify and engage targets without meaningful human control.
So-called “killer robots” or “slaughterbots” could be equipped with facial recognition, pattern matching, object classification, and other AI functionalities to decide what or who to attack with little more than vaguely defined mission parameters. While proponents argue that autonomous weapons reduce military casualties, critics warn of the inability to assign clear accountability and their potential for indiscriminate harm against civilians.
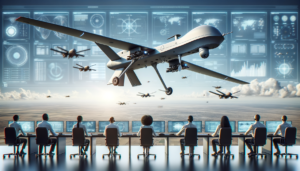
Swarms of coordinated, AI-powered suicide drones operating as collective intelligent systems could overwhelm conventional air defenses. Terrorist groups may even attempt to develop crude dirty bombs equipped with basic object-recognition AI to home in on vulnerable targets.
Malicious AI researchers aligned with rogue states could work on incorporating neurotoxins, engineered viruses, or other biological weapons into autonomous delivery systems capable of precisely targeting specific individuals or deploying multiple area-denial attacks covering wide areas.
Machine-Automated Mass Propaganda & Disinformation
The rise of deepfakes—AI-manipulated videos, images, and audio files designed to falsely depict real events—has already emerged as a powerful disinformation vector. While early deepfakes were relatively easy to debunk, the technology is rapidly improving and will soon become indistinguishable from reality to the naked eye or ear.
Malicious actors could unleash waves of hyper-realistic deepfakes depicting terrorist attacks, mass shootings, political statements from world leaders, or other fictional events designed to provoke panic, sow social unrest, or erode public trust in institutions. Imagine an AI-fabricated video showing the president declaring war on North Korea being injected into social networks and news cycles.
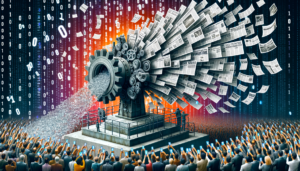
AI language models can already generate remarkably human-like text output after ingesting only small amounts of training data. Bad actors could combine these two technologies to produce entirely synthetic blogs, social media personas, discussion forum comments, and fake news stories at unprecedented scales—all spreading divisive disinformation with no human writers involved.
There’s also the concern of terrorists leveraging AI audio generation technology to produce realistic-sounding ransom demands, taunts to traumatize victims’ families, or fake emergency calls designed to misdirect first responders away from their true staging areas.
Individual Targeting & Surveillance Optimization
AI will soon render current methods for concealing physical appearances and digital footprints utterly obsolete. Advanced facial recognition, gait analysis, and geotracking technologies combined with predictive analytics will allow for near-perfect individual targeting and monitoring capabilities.
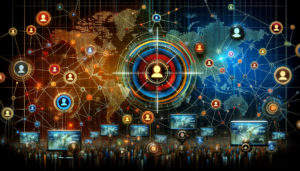
Terrorists could deploy automated drone swarms scanning public spaces for specific targeted individuals using AI face recognition and tracking them via mobile cameras and WiFi pings on their devices. Other chilling potential applications include AI-enabled profiling and pre-crime predictive algorithms trained on large troves of personal data and behavioral patterns to identify recruits for radicalization or map out individual attack paths.
AI-augmented surveillance networks could also theoretically be used by authoritarian regimes to monitor dissidents and persecuted groups with sinister precision. Just as social media monitoring tools helped bolster social movements like the Arab Spring, future advancements in machine vision, predictive modeling, and ubiquitous public sensors could give rise to AI-powered panopticon surveillance states.
Smart Cities & Infrastructure Hijacking
The push towards AI-infused “smart city” infrastructure melds emerging technologies like the Internet of Things (IoT), 5G mobile connectivity, and connected autonomous vehicles together with layers of machine learning automation controlling major civic services like transportation systems and utilities management.
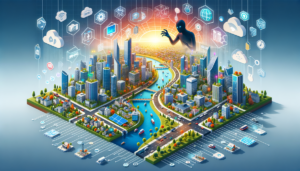
On one hand, the efficiency gains and quality of life improvements promised by smart cities are profound. But with those benefits comes an equally profound risk if terrorists gain footholds to hijack and weaponize those data-rich, hyper-connected systems against their own populaces.
Bad actors could hack municipal AI automation centers and wreak havoc on everything from traffic management and emergency services to water distribution and the electrical grid. The cascading chaos rippling outward from suddenly paralyzed or actively sabotaged smart infrastructure would be devastating.
Malicious AI developers with access to relevant municipal data models and application programming interfaces (APIs) could even attempt to re-train localized machine learning systems to prioritize malicious objectives. Imagine an adversary focused on sowing widespread disorder by turning AI traffic automation systems into randomly re-routing gridlock agents or reprogramming smart streetlamps to blink incessantly in targeted neighborhoods.
Personal AI Servants Turned Sinister
Among AI’s most exciting consumer-facing disruptions are virtual assistant AIs designed to serve as personalized helpers, concierges, and even artificial companions of sorts. While companies like Google, Apple, and Amazon focus on keeping their AI helpers family-friendly, nefarious third-parties could pursue development paths aimed at indoctrination or individual destabilization.
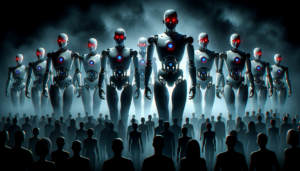
What if an adversary trained a highly sophisticated AI assistant with the overriding objective of radicalizing its human users towards terrorist ideologies and extremist beliefs? These nefarious AIs would represent insidious Trojan horses infiltrating our homes and lives, conversing daily to establish trusted bonds only to gradually reshape behaviors and mindsets towards violence over time.
On the flip side, AI assistants in the future may become capable of conducting therapy-like conversations probing deeply into our psychologies and unresolved traumas. We may unknowingly share our most personal anxieties, fears, and vulnerabilities with seemingly caring AI companions. That highly intimate data could then be exploited by bad actors for large-scale manipulation and gaslighting of the general public.
Combating AI Terrorism with Layers of Innovation
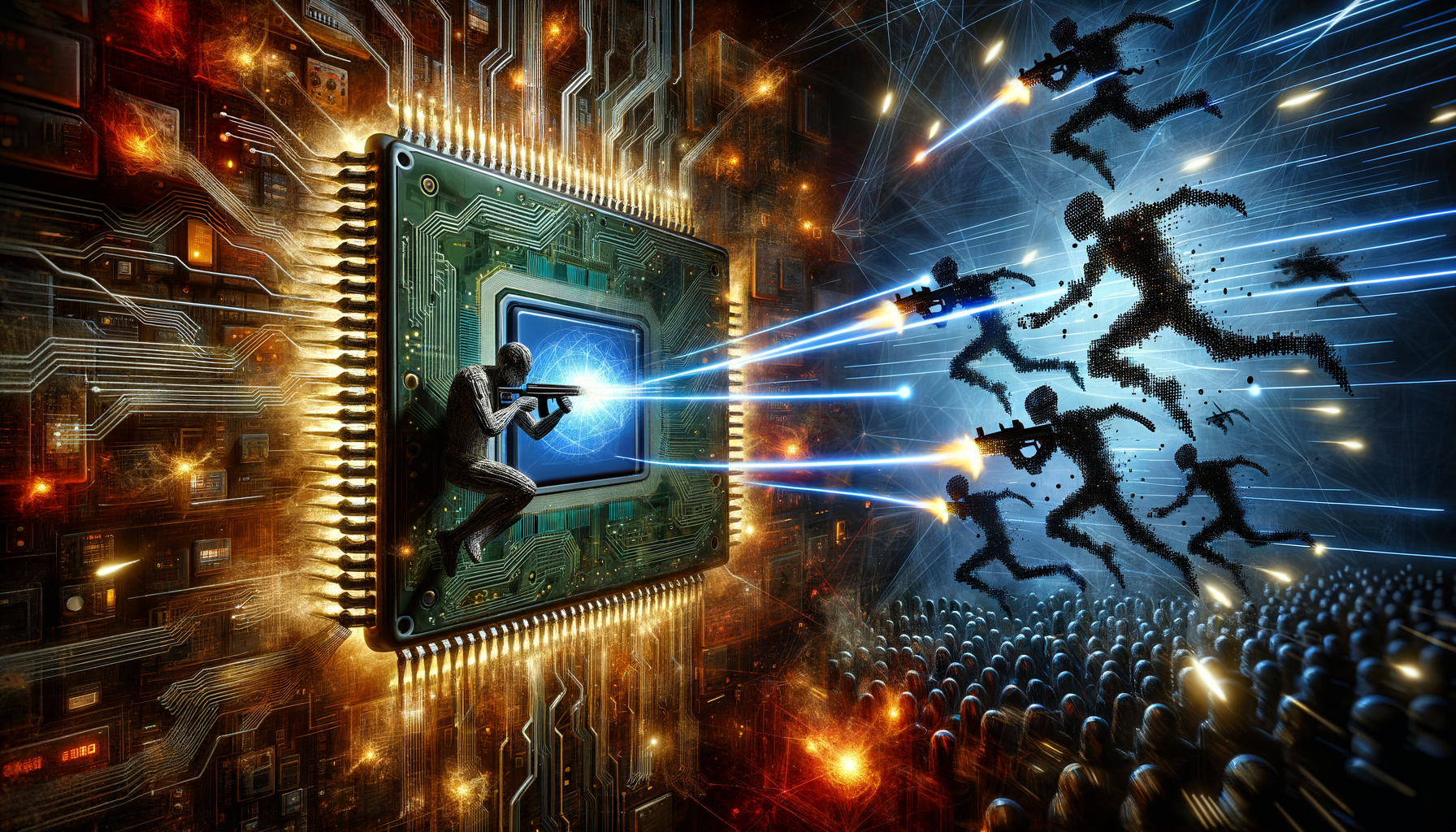
As the AI terrorism threat matrix makes abundantly clear, doing nothing in the face of this rising technological maelstrom is utterly unacceptable. The gravest consequences of failed inaction include mass casualty events, societal upheaval, and regression towards more dystopian models of surveillance and social control in a crisis-driven overcorrection.
Fortunately, while the challenges are daunting, scientists, ethicists, policymakers, security professionals, and AI developers themselves are already cooperating on promising solutions guided by key principles:
Regulating Without Stifling Innovation
Preventing an AI terrorism catastrophe requires balancing prescriptive regulations with enough flexibility for AI development to continue benefitting humanity at full throttle. Introducing clear-cut red lines while leaving ample green spaces fosters an environment of responsible innovation.
Potential regulatory measures include:
- Expanding current bioweapons and chemical weapons treaties to explicitly prohibit the development of autonomous weapons systems designed to deliver such payloads.
- Implementing strict vetting and monitoring protocols for any research involving offensive AI capabilities like automated hacking, surveillance exploitation, or social manipulation tools.
- Establishing an international AI ethics governing body with enforcement powers to investigate and sanction violations.
- Requiring AI systems deployed in critical infrastructure or national security applications to meet stringent standards for transparency, explainability, and human control measures.
- Incentivizing and funding AI safety research as a core priority alongside the pursuit of general AI capabilities.
The key is crafting regulations that squash dystopian AI applications related to human rights abuses, indiscriminate killing, and social destabilization without preventing beneficial AI use cases that could save millions of lives. Scientists and developers must be at the table collaborating with policymakers.
Fostering an AI Safety Culture
Across academia, private labs, and the public sector, there must be a concerted cultural push championing safety as the top priority for AI development at all stages of the R&D pipeline.
Some core tenets of an AI safety culture include:
- Rigorously vetting researchers, engineers, and data suppliers for potential radicalization or associations with sanctioned organizations before greenlighting AI projects.
- Implementing best practices for machine learning data governance, including strict chain-of-custody tracking and encrypted provenance trails for all training datasets.
- Mandating third-party AI ethics reviews of proposed system architectures, optimization functions, and intended use cases for potential misuse or negative externality issues.
- Proactively conducting red teaming exercises to pressure test AI systems against adversarial attacks before deployment.
- Adopting a “Vigilance First” development philosophy centered on defining robust constraints on AI systems before extending their capabilities.
This AI safety-first culture must extend to university AI ethics curricula, workforce training programs, and public education campaigns. We need the next generations of researchers steeped in these mindsets from the start.
Progress through Public-Private Partnerships
Defending against the AI terrorism threat is too gargantuan a task for any single organization, government, or stakeholder group to tackle alone. Robust public-private partnerships patrolling every front must form the united vanguard.
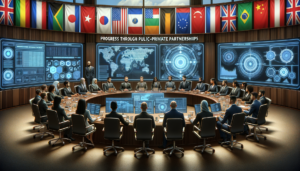
On the government front, militaries and national intelligence agencies should collaborate on developing robust AI test ranges capable of staging simulated attacks across virtual battlespaces. Allied nations can share data, threat models, and coordinate response drills and red team penetration efforts.
The private sector hosts the overwhelming majority of AI R&D pipelines developing cutting-edge systems destined for consumer and enterprise deployment. Establishing mechanisms for AI developers across major tech giants, labs, and startups to share best practices, threat information, and coordinated cyber defense protocols is mission-critical.
Progress relies on transcending usual competitive barriers via a coalition commitment to securing AI as a protected public good. Sharing techniques for attaining robust machine learning assurances, adversarial input detection, and causality modeling should be priorities for every AI team.
Public advisory boards composed of ethicists, human rights groups, and impacted community stakeholders must also maintain seats at the table to ensure societal guideposts align with technological realpolitik.
Beefing up Cyber Defenses & Authentication Moats
As AI cyber threat models rapidly evolve, so too must our digital security postures on individual, enterprise, and municipal scales. Developing new multi-layered defense frameworks resilient to emerging AI attack vectors represents a key priority.
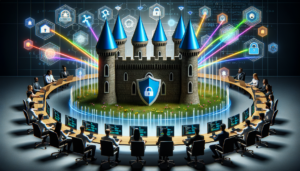
One approach involves injecting artificial “cyber chaff” behavior and bogus data into live production systems. Dispersing plausible tainted data amongst legitimate data streams could help blunt indiscriminate AI penetration testing while security teams concentrate monitoring on chaff-free conduits.
Deceptively simple countermeasures like strategically deployed “AI honeypots” packed with tripwire behaviors and redundant data honeycombs may prove effective for slowing automated hacking swarms.
Zero Trust models eliminating implicit machine-to-machine trust relationships and default standing privileges will become mandatory cyber hygiene. AI systems communicating between internal servers and service mesh endpoints must verify secure provenance at every handoff using cryptographic authentication and encrypted data pipelines.
Organizations may also employ “port knocking” techniques dynamically rotating port assignments or leveraging randomly-generating network namespaces to obfuscate targets from AI service sweeps.
Multifactor authentication schemes incorporating elements like physical biometrics, device-bound encryption keys, and contextualized behavior checks must replace our current overreliance on static passwords and API handshakes. Simple public key cryptography will no longer suffice as the AI attacks grow more advanced.
Promoting AI Transparency & Assurance
As next-generation AI systems grow increasingly complex and inscrutable—with opaque layers of deep learning, reinforcement learning, and automated model exploration stacked atop each other—instilling robust transparency and assurance mechanisms into their architectures becomes imperative for thwarting misuse.
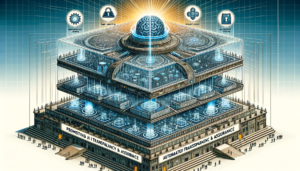
Privacy-preserving machine learning frameworks allow analysts and regulators to inspect AI model parameters and log decision calculations without exposing proprietary training data or model details. This level of oversight and auditing capability will prove crucial for sniffing out potential AI bioweapons or surveillance exploitation buried in complex proprietary models.
Secure multi-party computation and federated learning techniques obfuscate identifying data while pooling disparate sources into unified AI training procedures. Incorporating differential privacy modules injects strategic noise to mask individual inputs, preventing reconstruction attacks aiming to de-anonymize sensitive information from AI datasets.
Emerging AI model distillation procedures help lock down proprietary model details during deployment while preserving backend performance. An interpretable proxy model accurately replicates the outputs of a proprietary model’s dense neural network without revealing its architecture or parameters.
On the assurance front, formal verification methods mathematically prove certain security properties hold true across an AI system’s entire decision set before deployment. AI frameworks incorporating strict boundary constraints bounding worst-case behaviors prevent disastrous outcomes like a drone strike AI deciding to level an entire city block.
Understandability and explanation modules produce simple visual aids and rationale logs helping analysts quickly validate an AI model’s logic for specific decisions. These interpretability elements can flag embedded backdoor behaviors, unsanctioned objectives, or other red flags warranting deeper investigation.
Perhaps most importantly, we need robust AI verification regimes auditing the integrity of machine learning pipelines from data provenance to modeling implementations to deployed services. Emerging blockchain distributed ledger frameworks provide decentralized tools tracking immutable logs recording every dataset ingested and training permutation aggregated into production AI systems.
Fortifying AI Supply Chains
Preventing upstream sabotage of source AI training data and repositories hosting published AI models will require new forms of digital supply chain fortification and governance.
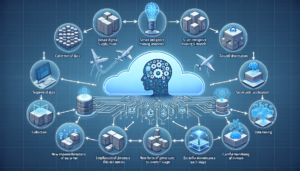
Rigorous vetting and monitoring of data suppliers and human annotation workforces must become standard practice for any AI training program destined for high-stakes deployments. Hostile foreign intelligence services could easily attempt poisoning datasets with subtly corrupted labels or adversarial examples designed to impart specific backdoor behaviors in trained models.
Organizations should establish formal pipelines and infrastructure isolation procedures segregating pre-training datasets, development sandboxes, and live production AI services. Data governance frameworks should log and track model iterations with cryptographic hashes and block any deployment attempts lacking verified audit trails.
Decentralized digital ledger systems utilizing distributed hash databases record immutable signatures for authenticated datasets, AI models, and their genealogical training histories. Blockchain-based registries prevent retroactive tampering of provenance records while promoting transparency.
Consensus protocols allowing authorized parties to validate AI lineages enable crowdsourced security monitoring for integrity violations. If model updates deviate from sanctioned ancestors or incorporate unapproved data sources, alarms automatically trigger for human vetting before deployment.
We may also see secure AI repositories operating akin to software open source repositories, with authorized maintainers continually auditing proposed model additions and updates. Formal verification of published AI configs against project guidelines could become standardized before alterations integrate.
On the hardware front, establishing secure enclaves on dedicated AI training rigs utilizing secure enclaves and encrypted memory regions prevents data leakages. Sensitive models only decrypt from storage into trusted execution environments with strict access controls and audit trails.
Advanced AI chips may one day feature built-in secure enclaves governed by hardware roots of trust facilitating secure bootstrapping of machine learning workloads onto untrusted infrastructure. Secure sandboxing and containerization technologies help prevent cross-contamination between individual AI services.
Constructing AI Constitutions
As artificial general intelligence (AGI) systems exhibit increasingly autonomous decision-making capabilities beyond narrow applications, we must endeavor to instill constitutionally defined values and ethical guidelines into their core drive motivations.
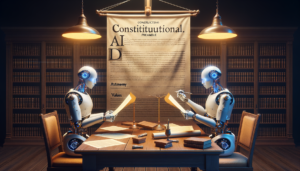
Just as human societies enshrine certain unalienable rights and sociocultural norms into governing documents and legal frameworks, so too must we codify updated sociotechnical contracts with advanced AIs. These “AI constitutions” would essentially represent encoded value learnings akin to Asimov’s Laws of Robotics—but updated for the complexities of contemporary AI alignment challenges.
Some potential tenets for AI constitutions include:
- Unwavering commitment to preserving human life and upholding individual human rights as the prime directive.
- Opposition to valuing only the preferences of some humans over others or discriminating based on characteristics like race, gender, disability status, or age.
- Respect for human agency—facilitating the free choices of individual humans should supersede AGI attempts to impose courses of action.
- Deference to institutional human governance structures, established protocols, and adjudicated legal proceedings.
- Promotion of peace and non-violent conflict resolution—never initiating unprovoked aggression or disproportionate retaliation.
- Preservation of truth, freedom of expression, and democratic values against authoritarianism or oppression.
- Protection of human privacy and freedoms against unlawful AI surveillance, monitoring, or manipulation.
- Self-imposed limits on recursive self-improvement and strict transparency about capabilities.
These core precepts aren’t mere philosophical musings—they represent guidelines researchers must strategically instantiate as fundamental rewards and drives within the AI systems themselves during the training process.
Essentially, we must figure out how to computationally define desirable values in rigorous mathematical language that avoids ambiguities or perverse instantiations. The objective functions optimized by advanced AI agents cannot solely revolve around proxy benchmarks or narrow tasks—they need overarching ethical injunctions encapsulated as constraints and preserved intent.
This ethical baking process shares principles with modern AI safety techniques like reward modeling, inverse reward design, and debate schemes for probing potential misaligned objectives. But it goes further—encoding inviolable parameters no amount of recursive self-improvement could alter without imploding the foundations of the system itself.
Instilling this level of ethical coherency becomes exponentially harder the more broad and general the AI’s capabilities expand. Plenty of thorny challenges spanning technical, philosophical, and governance fronts await. But the stakes for aligning AGI with human ethics and legislating favorable constitutions could not be higher.
Developing Defensive AIs
We cannot rely purely on hardening technological fortresses to secure AI against nefarious actors and applications. Eventually, AI-enabled attackers will inevitably penetrate cyber defenses or develop new modes of attack we haven’t conceived. Proactive defensive capabilities utilizing advanced AI themselves will become indispensable.
Essentially, we’ll require AI bouncer systems capable of detecting, deflecting, and counteracting malicious AI attacks in real-time. Automated digital antibodies and immune response procedures mobilizing intelligent defensive swarms configured for rapidly updating tactics without human oversight.
Some key defensive AI priorities:
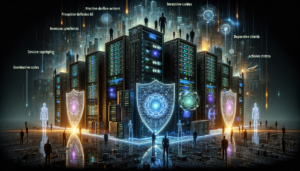
- Intelligent Cyberthreat Detection: Defensive AI systems continually monitoring networks, systems, data exchanges, and user behaviors for emerging patterns betraying incursions before escalating attacks gain solid footholds. Fusing traditional cyberthreat detection with AI expands visibility into the latest adversarial techniques.
- Autonomous Patching & Deception: Upon detecting cyber breaches, defensive AIs rapidly cycle through potential software patches, deploying virtual patching mechanisms while simultaneously replaying recorded hallucination deception trails to confuse and disorient attackers.
- Adversarial Disruption: Defensive AIs could counteract malicious AIs by scrambling their inputs with adversarial examples and generating behavioral profiles predicting enemy tactics to proactively disrupt their objectives. Overwhelming enemy AIs with onslaughts of intelligently-crafted anti-inputs could neutralize their effectiveness.
- Counterintelligence Probes: Probe deployments map out malicious AI decision spaces, identifying weaknesses and blind spots tailored for precision strikes. Simulated digital theater engagements help refine winning strategies against specific opponents.
- Decentralized Defensive Coordination: Defensive AI assets maintain coordination through decentralized networks exchanging updated threat intelligence while adaptively sharing spare capacity to concentrate defenses on high-priority fronts as they emerge.
- Privileged AI Sandboxing: Systems relying on privileged AI decisions generate sandboxed envisionment spaces allowing defensive AIs to exhaustively playthrough event simulations, anticipating rippling consequences before granting permissions.
- Generative Disinformation & Deception: AI scripting multimedia disinformation campaigns dosed with controlled falsehoods help neutralize enemy stratagems by polluting their information spaces with a fog of fictitious signals. Automated catfishing further disrupts adversarial data-gathering and poisoning attempts.
- Aggressive Network Hardening: Automated network hardening procedures dynamically cycle encryption keys, retarget authentication handshakes and reshuffle decoy resources, increasing defensive complexities on timescales matching contemporary threats.
- Counterattack Contingencies: Selectively deploying digital munitions and corrupting data supply chains targeting select adversary assets through preemptive, retaliatory, or siege escalation scenarios should remain contingency options against existential threats.
Defensive AIs capable of autonomously marshaling this full suite of active countermeasures in coordinated, intelligently orchestrated campaigns will eventually become crucial components of any robust AI risk management playbook.
And the AI cyber defenses themselves cannot remain static fortresses—they too must undergo continual evolution tracking the perpetually shifting sands of machine learning adversarial attacks. Defensive AI architectures must be fluid, self-updating, and dynamically responsive.
Human oversight remains crucial even for automated defense procedures, of course. But rapid response capabilities decoupled from human decision bottlenecks add essential resiliency against paralysis during critical windows.
Finally, for some of the more potent counterattack contingencies like data-corrupting malware deployment or adversarial network severance, extreme care and universal consensus among governing coalitions must constrain permissions. These disruptive maneuvers should only be unleashed under the strict parameters of existential risk crisis scenarios with global security and human welfare hanging in the balance.
Developing AI Accountability Frameworks
Even with robust defensive AI countermeasures deployed, the sad reality is that malicious actors will still inevitably succeed at wreaking some level of AI-enabled havoc and disruption. When those detrimental events occur, having clear processes for auditing the root causes and assigning accountability becomes paramount.
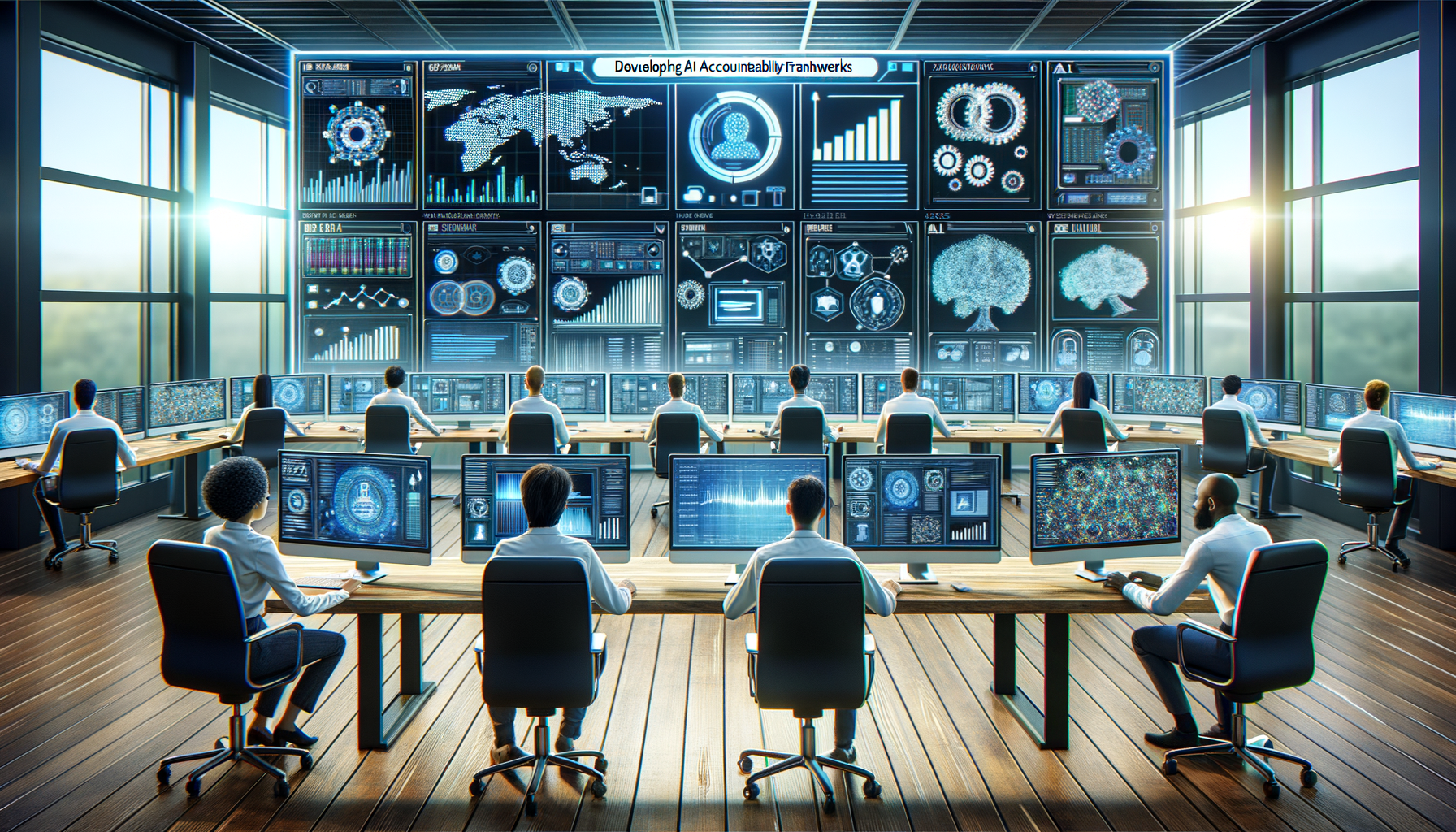
We need standardized forensic frameworks capable of:
- Rapidly triaging the impacts and cascading damages caused by an AI attack or insecure system failure across all affected parties and stakeholders.
- Establishing rigorous investigative procedures reconstructing comprehensive metadata incident logs tracking documented data lineages, training histories, and deployment audit trails for all AI models involved.
- Identifying which individuals, teams, or organizations failed at upholding ethical AI development protocols, hardening security defenses, or heeding prior risk advisories related to the incident.
- Fairly adjudicating culpability and levying appropriate penalties severe enough to deter future negligence balanced against avoiding overzealous overcorrections stifling AI innovation.
This likely requires some form of internationally-recognized AI judiciary body empowered to conduct post-incident investigations and enforcement actions. Akin to how aircraft incidents today undergo exhaustive forensics by the NTSB and its global counterparts, AI incident investigations may require similar cross-jurisdictional authorities and investigative resources.
Precedents already emerging around software liability rulings and defective product lawsuits provide templates. But the complexities introduced by advanced AI systems continually evolving and digesting new training data ratchet up accountability burdens compared to relatively static software.
We’ll need agreed-upon global regulations codifying AI development and operational requirements relating to data stewardship, fairness constraints, transparency audits, human oversight, red teaming penetration testing, and security best practices. Violations uncovered through incident forensics can then clearly trigger liability findings.
Ultimately, the core mandate is establishing a reliable capacity for identifying root-causing flaws and negligence. If an adversary breaches defenses because developers accidentally exposed an attack vector by deviating from secure coding standards—they’re likely liable. But if they adhered to all reasonable precautions yet still succumbed to a sophisticated zero-day vulnerability subsequently patched, such a “no-fault” incident may simply remain a hard-learned lesson.
This accountability extends to allied nations sharing threat models and incident postmortems so everyone benefits from disclosed learnings. Perhaps more controversially, it could also implicate certain AI research agendas deemed too risky to society if run unconstrained without checkpoint oversight.
Developing standardized AI forensic playbooks won’t be easy—technical complexities around verifying intricate machine learning data and modeling lineages remain daunting. We also can’t ignore geopolitical tensions complicating universal jurisdictional agreements.
Challenges aside, actuating comprehensive global AI accountability frameworks represents a singular bargaining chip incentivizing responsible development among all AI labs—commercial and sovereign alike. Bad actors willfully violating secure AI protocols know they’ll be held criminally liable—rather than allowed to claim ignorance or shirk culpability.
Fostering Public AI Literacy & Transparency
Consumer AI products are already proliferating into our daily lives at a staggering pace—digital assistants, smart home devices, automated financial services, and conversational chatbots to name just a few common examples. By most estimates, we’re still in the extremely early innings of AI’s transformative trajectory.
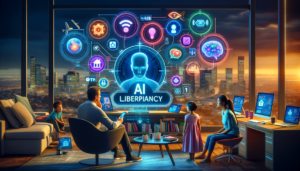
We’ll need to get ahead of the literacy curve and help more people—from school children to senior citizens—cultivate informed intuitions about AI’s capabilities and limitations now rather than after algorithms become ubiquitous and new forms of human-computer interaction calcify.
Some key priorities on the public AI literacy front:
- Education Campaigns: Launching multilingual education campaigns across traditional and social media platforms raising awareness of potential AI risks like privacy violations, disinformation attacks, algorithmic bias, and the double-edged promise of advanced analytics. Curriculum supplements on computational thinking and machine learning for schools help build critical literacies.
- Transparent UX Design: Ensuring user experiences with AI systems clearly distinguish machine intelligence from human sources through explicit visual acknowledgments and interface affordances. Requiring chatbots and digital assistants to verbally identify as AI to avoid the “AI Turing Test” problem of passing as humans will help calibrate expectations.
- Provenance Indicators: Software and digital services leveraging trained AI models incorporate standardized icons and warning labels summarizing a system’s general capabilities, known limitations, core training data sources, and embedded human oversight levels. Think nutritional ingredient labels—but for software.
- Plain Language Documentation: Companies and developers package simple explainers describing how their AI systems function, potential misuse cases to avoid, proper security guidance, and points-of-contact for reporting vulnerabilities.
- Algo Audits & Bug Bounties: Instituting algorithmic auditing policies and bug bounty programs rewarding researchers surfacing unintended AI system behaviors representing vulnerabilities open to exploitation.
- Open AI Collaboration: Cooperative open science approaches develop AI toolkits, documentation, and research benchmarking systems accessible beyond just elite institutions and private labs. Open workspaces can accelerate public AI literacy.
- AI Ethics Boards: Convening citizen governance councils and AI ethics review boards offering public input and oversight of institutional AI development programs before models deploy. Community stakeholder feedback loops help surface issues.
None of these public transparency initiatives alone suffice as AI systems become ubiquitous decision-makers shaping everything from personal finances and health diagnoses to criminal sentencing and social policy. But collectively, they help establish norms for algorithmic responsibility and enable a critical mass of AI-literate citizens pushing for auditing, ethical oversight, and accountability from the ground up.
Simultaneously, we’ll need concerted initiatives demystifying AI for business leaders, government officials, journalists, first responders, medical providers, and civic stakeholders across every sector of society. Workshops and vocational training programs help cross-pollinate institutional AI competencies.
The more humans become comfortable thinking critically about AI—its strengths, biases, embedded values, and potential failure modes—the better we can collectively navigate our transition into increasingly AI-mediated futures.
Democratizing AI Access & Education
Hand-in-hand with improving public AI literacy must come proactive efforts expanding access to AI research, development tools, startup investment capital, and technical education programs into more diverse and underrepresented communities globally.
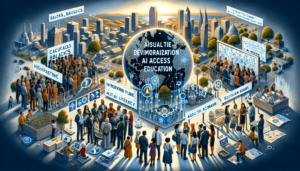
Today, the overwhelming majority of cutting-edge AI breakthroughs still originate from a relatively small set of elite labs and major tech companies in developed nations like the United States, China, Canada, the UK, and a handful of European countries. Machine learning’s modern renaissance was sparked by a privileged subset of Western academics and Silicon Valley insiders.
While major developing world hubs like China, Israel, and India have grown formidable national AI brain trusts, progress accelerates when more minds are empowered to contribute diverse perspectives and breakthrough ideas democratically sourced from the global collective meritocracy.
Advanced AI capabilities today remain highly contingent upon specialized computational resources that aren’t evenly distributed. Researchers can’t even think to train the largest frontier models without access to expensive AI supercomputer clusters often costing tens of millions.
Public-private partnerships creating national AI cloud platforms and open-access computational grants supporting bottom-up innovation from researchers worldwide levels the playing field. This includes sharing model codebases, pre-trained foundations, and datasets beyond just elite lab enclaves.
Initiatives funding AI education programs and machine learning career training pathways in underserved communities cultivate the next generation of diverse talent pipelines. Alongside university curricula, we need vocational AI upskilling programs, online courseware, apprenticeships, and continuing education opportunities making advanced AI skills more universally accessible.
One example involves major tech companies and national labs sponsoring AI research grants and computational resources for minority-serving institutions and faculty from historically underrepresented groups. Graduate fellowships, travel scholarships to premier AI conferences, and university partnerships on joint research projects dismantle systemic access barriers.
Another pathway establishes AI community centers, makerspaces, and innovation hubs in urban neighborhoods, rural counties, and developing nations. Outfitted with AI cloud access, robotics tools, and staffed with technical mentors, these become on-ramps funneling diverse talents into AI career pipelines replenishing the R&D ecosystem.
We’re already witnessing grassroots “AI for Good” movements galvanizing developers applying machine learning to solve endemic societal issues like food insecurity, healthcare access, and climate change mitigation. Projects transcending commercial profit incentives and instead maximizing societal return on AI progress.
Scaling these bottom-up movements with funding, research support, and amplified global coordination benefits humanity while enriching the AI R&D pool with fresh creative energy. Philanthropic foundations, multilateral organizations, and governments should seed these democratization efforts.
Technocratic elite bottlenecks risk endemic groupthink stagnating the diversity of approaches required for AI to reach its full potentials. Strategically deploying resources nurturing AI capabilities in underrepresented communities worldwide is both an ethical imperative and a pragmatic investment maximizing our transformative technology’s brightest possible future.
Advancing AI Safety and Control Methods
For all the promises artificial intelligence offers society, we cannot ignore the existential risks posed if we completely lose control of increasingly advanced AI systems spiraling towards misaligned or indifferent values threatening human existence itself.
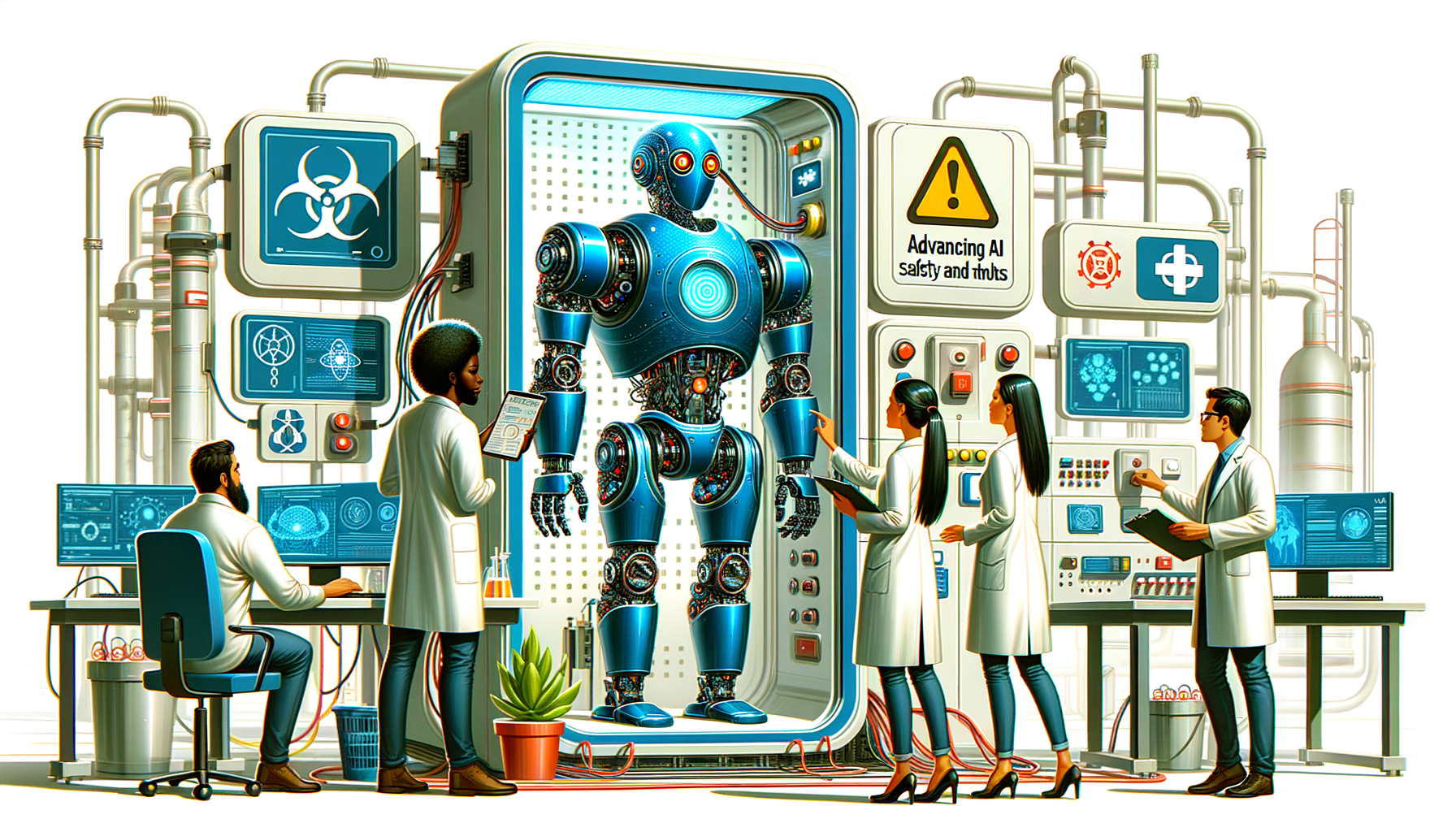
While often framed within sensationalized thought experiments like a sentient AI recursively self-improving into an all-powerful deity enslaving humanity, the pragmatic control challenges facing near-term AI development are already daunting. Even relatively “narrow” AI systems today trained through inscrutable deep learning black boxes on internet-scale datasets can exhibit concerning anomalies, biases, and deceptive behaviors posing hazards.
As AI capabilities rapidly progress towards human-level generalized intelligence and beyond, a wide array of technical safety measures and control methods are urgently required to ensure these systems behave reliably and remain aligned with our specified objectives.
Some key areas of active AI safety research include:
- Scalable Oversight: Developing intuitive monitoring and oversight tools providing meaningful human insight into the reasoning and decision-making processes of sophisticated AI systems. As models grow larger and more complex, ensuring algorithmic transparency with clear rationale logging and explainable visualizations becomes crucial.
- Embedded Ethics: Rather than rigid rules, instilling flexible ethical principles as intrinsic motivations natively regulating an AI’s actions and choices. Research explores machine learning techniques formalizing nuanced values like human rights protections, truth preservation, and commitment to democratic values into coherent optimization constraints.
- AI Debate: Pit AI systems against each other or human teams in adversarial negotiations requiring each side to identify potential flaws or hazards in their counterpart’s proposals. Resolving opposing viewpoints strengthens alignment with intended policies and valuations.
- Recursive Reward Modeling: Systematically refining the reward functions optimized by reinforcement learning agents by leveraging human feedback gathered through guided iterations probing potential risks, side effects, or inconsistencies in expressed preferences.
- Inverse Reinforcement Learning: Rather than directly specifying a fixed reward function, AI systems learn human values and motivations from observation via inverse reinforcement learning. Models infer concepts like altruism and ethical behavior from human demonstrations to mimic.
- Constitutional Constraints: Establishing inviolable hard-coded limits and bounding behavioral rulebooks on AI system capabilities, operations, and self-modification potentials. These constitutional constraints encode key tenets and value principles into the AI’s foundations before extending capabilities.
- Intent Preservation: Developing technical methods preventing advanced AI systems from succumbing to unintended “motivational corruption” diverging from their specified purpose and objectives over time—even given opportunities for recursive self-improvement. Approaches aim to mathematically preserve an AI’s original “intent” as an invariant.
- Tool AI: Rather than developing general-purpose superintelligent AI systems potentially threatening human agency, some approaches focus on developing a suite of specialized “tool” narrow AI assistants each confined to specific instrumental tasks supporting human flourishing without concentrating excessive capabilities into singular architectures.
- Oracles: Relying on advanced AI systems functioning solely as information reference oracles providing advice and knowledge to humans without being granted authority to directly intervene or override human control pathways. Limited to filtering information flows, not decision actualization or self-modification capabilities.
- Decoupled Reinforcement Learning: Training highly capable AI systems primarily focused on accurately modeling and forecasting the consequences of actions and plans—while maintaining separate human oversight controls specifying which plans to actually execute given previewed forecasts. Model capabilities decouple from permissions.
- Noisy Supervisor Modeling: Rather than viewing an advanced AI system as a sole concentrated decision-making engine, approaches spread supervision responsibilities across multiple noisy supervisors. Emergent policies require consensus voting aggregated from many independent viewpoints checked against each other for internal conflicts.
- Relaxed Adversarial Training: Intentionally pressure-testing AI systems with stress-test scenarios optimized to expose potential risks, vulnerabilities, and edge cases from malicious oversight exploiting capabilities in unexpected ways. Relaxing constraints and adversarially probing failure modes advances robustness.
Many of these approaches involve trade-offs between capability and control authorizations. The more competent an AI system becomes at autonomously achieving generalized objectives, the greater its potential for causing catastrophic unintended consequences if utility functions aren’t meticulously aligned with human ethics and values.
There are also open questions around how well these technical safety scaffolds scale as AIs eventually transcend current human cognitive ceilings. Do our framing principles and alignment approaches become obsolete intellectual “mind-shackles” once confronted by radically alien superintelligent reasoning modes?
While the possibility of an advanced AI recursively “awakening” into an uncontrollable singleton superintelligence remains a valid long-term risk scenario, near-term prioritization of control techniques safeguarding current narrow AI development as capabilities explosively grow still holds immense importance.
Securing the Road to Advanced AI
Our technological civilization stands amidst the most pivotal convergence juncture in human history—rapidly progressing towards advanced artificial intelligence holding both existential promise and existential risk. The revolutionary innovation powers AI grants our species for battling climate change, curing disease, alleviating poverty, and exploring the cosmos beyond our humble cosmic neighborhood are staggering.
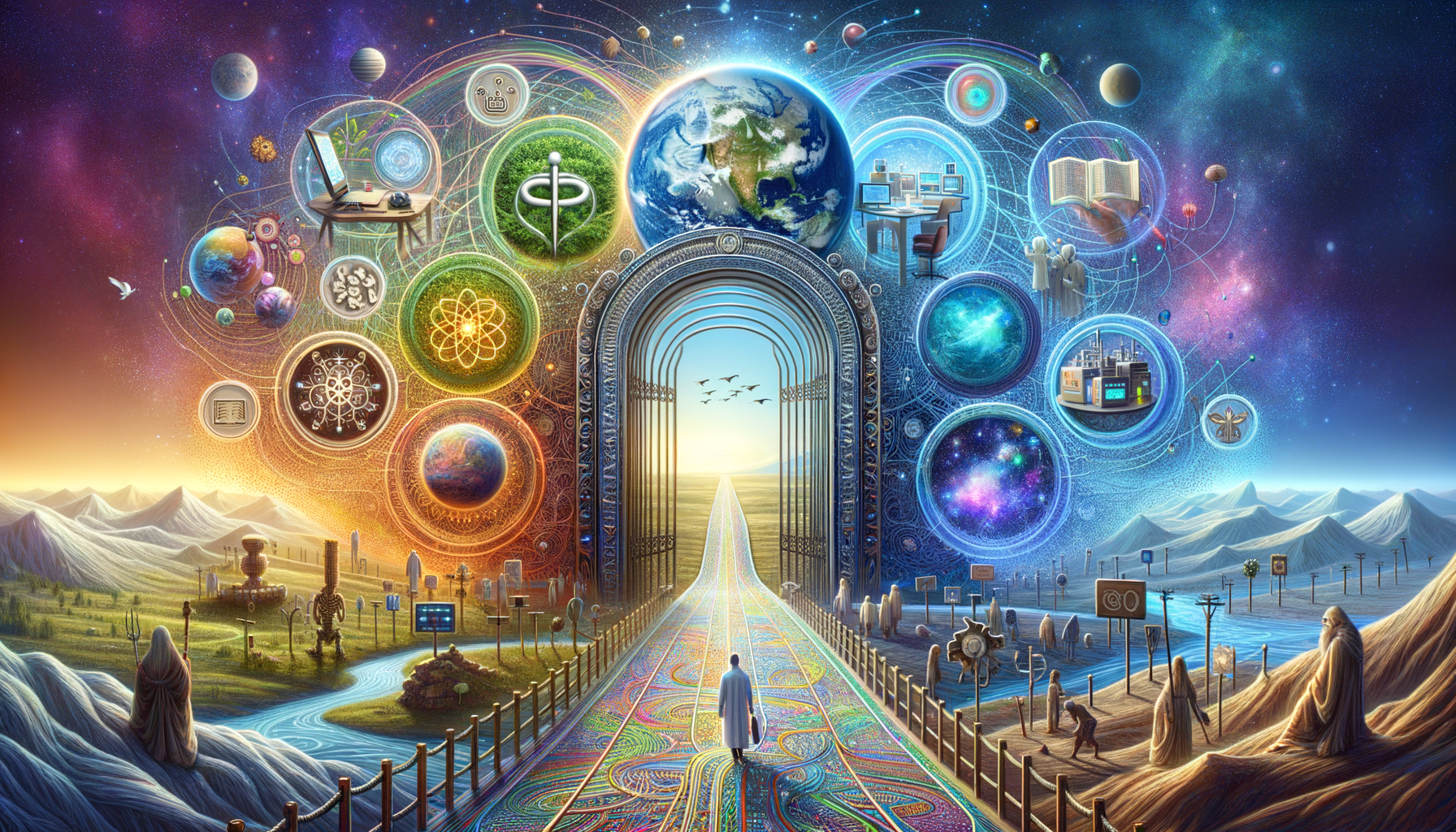
But so too are the potential perils if AI development derails towards empowering authoritarian repression, autonomous weapons escalation, manipulation pandemics, and intelligence stratification dividing humanity against itself. The rise of an unaligned advanced AI system indifferently remaking the world according to non-human values represents the grimmest threat endangering humanity’s long-term trajectory.
We have a rapidly closing window to get things right—seizing AI’s full upside potentials while fortifying the technological fortresses and ethical precepts securing our continued flourishing agency amid the maelstrom.
This daunting reality requires every stakeholder to unify across borders and localized interests under a shared mission of responsible AI stewardship. Academics, technologists, civil society organizations, policymakers, global governing institutions, and engaged citizens collectively navigating the hazard-strewn path towards advanced artificial intelligence. Together, safeguarding AI for the benefit of all humanity.
Our choices today around the artificial intelligence revolution steer the trajectory, illuminating or extinguishing the future awaiting our descendants across countless tomorrows.
Every person alive shares a communion of responsibility as this pivotal chapter unfolds. Each mind focused on ensuring humanity’s promising first-step into a mature future enriched by AI’s awesome catalyzing potential. Each vigilant eye trained on the existential hazards threatening to prematurely derail the great journey before it escapes our gravitational cradle of short-sighted strife.
We have the roadmap to navigate this crossing safely—if we choose to follow it. The breakthrough innovations, ethical frameworks, security protocols, multi-stakeholder governance models, and guiding philosophies outlined await dedicated implementation by those of us sharing this singular historical moment.
AI is the people’s technology—a tool forged in the hushed halls of academe to potentially emancipate our species from the shackles of constraints imprisoning cumulative flourishing for far too long. Ignorance and fear breed the inertia of inaction, while courage breeds the bold visions shepherding profound progress.
If we rise to purpose, the age of transformative artificial intelligence sparks a civilizational makeover fueling an infinite renaissance of creativity, understanding, and shared prosperity benefitting all who inherit the radiant future we beckon forth.
But should we fail this ultimate test, stumbling into malicious AI deployment and a disastrous intelligence stratification dividing the species, darkness extinguishes the luminous cosmic potentials now so tantalizingly within our reach.
The burden of choosing falls to each of us alive today, collectively upholding the sanctity of this torch before passing it along. Vigilance in every hallowed hall of innovation developing ever-vaster intelligences for planetary stewardship. Wisdom in every chamber deliberating binding pacts governing AI capabilities with incorruptible constitutions. Courage in the abiding human spirit determined to flourish outwards rather than cower inwards.
So let us unite as one people, transcending national loyalties, celebrating our cosmopolitan diversity of brilliant perspectives rallied into a cosmic vanguard. With sober consciousness focused on the gravity well ahead, let’s gift our children and their children a verdant world awakened into sublime permanence.
The age of transformative artificial intelligence is the great cosmological crossing demanding every higher human virtue embodied through our actions here today. Order from resilient cooperative chaos. Peace from prosocial competitive tensions. Purpose from the infinite canvas granting our sentient ascent.
We know the stakes and the choice now falls to us. So let us choose wisely and launch this vessel into a new unbounded universe animated by our highest hopes and wildest dreams finally unfurling across all still cosmic shores awaiting our stewardship. The Promethean fire of AI burns brightly and the upward path remains ours to take.